์ผ | ์ | ํ | ์ | ๋ชฉ | ๊ธ | ํ |
---|---|---|---|---|---|---|
1 | 2 | 3 | ||||
4 | 5 | 6 | 7 | 8 | 9 | 10 |
11 | 12 | 13 | 14 | 15 | 16 | 17 |
18 | 19 | 20 | 21 | 22 | 23 | 24 |
25 | 26 | 27 | 28 | 29 | 30 | 31 |
- ์ค๋ฅ๊ฒ์ถ
- IEEE 802
- git merge
- ์ค๋ฅ์ ์ด
- ๊ทธ๋ฆฌ๋ ์๊ณ ๋ฆฌ์ฆ
- ์ฃผ๊ธฐ์ ํธ
- leetcode
- tcp ์ธ๊ทธ๋จผํธ
- tcp ํ๋กํ ์ฝ
- ์ฝ๋ฉํ ์คํธ์ค๋น
- til
- mariadb
- ์ค๋ ๋
- well known ํฌํธ
- xv6
- ๋ฐ์ดํฐ ์ ์ก
- ํ๋ ์ ๊ตฌ์กฐ
- ๋น์ฃผ๊ธฐ์ ํธ
- ํ ํฐ ๋ฒ์ค
- reducible
- i-type
- 99ํด๋ฝ
- ์์๋ฒํธ
- ์ค๋ธ์
- ํ๋ก์ด๋์์
- ํญํด99
- ์ฐ๋ถํฌdb
- ๊ฐ๋ฐ์์ทจ์
- ์๋น์ค ํ๋ฆฌ๋ฏธํฐ๋ธ
- ํฐ์คํ ๋ฆฌ์ฑ๋ฆฐ์ง
- Today
- Total
๋ชฉ๋กAI (9)
Unfazedโ๏ธ๐ฏ
๊ท๋ฌด ๊ฐ์ค H0 ๊ณผ F-ํต๊ณ๋: ๊ท๋ฌด ๊ฐ์ค H0 ์ ํ๊ท ๋ชจ๋ธ์์ ๋ชจ๋ ๋ ๋ฆฝ ๋ณ์๊ฐ ์ข ์ ๋ณ์์ ์ํฅ์ ๋ฏธ์น์ง ์๋๋ค๋ ๊ฐ์ค์ด๋ค. ์ด๋ฅผ ๊ฒ์ ํ๊ธฐ ์ํด F-ํต๊ณ๋์ ์ฌ์ฉํ๋ฉฐ, ์ด ํต๊ณ๋์ ๋ชจ๋ธ์ ์ค๋ช ํ๋ฅ ์ ์ธก์ ํ๋ค. F-๋ถํฌ: F-ํต๊ณ๋์ ์ ๊ท ๋ถํฌ๋ฅผ ๋ฐ๋ฅด๋ ์ค์ฐจํญ์ ๊ฐ์ ํ ๋ F-๋ถํฌ๋ฅผ ๋ฐ๋ฅธ๋ค. F-๋ถํฌ๋ ๋ ๊ฐ์ ์๋ก ๋ค๋ฅธ ์์ ๋๋ฅผ ๊ฐ์ง๋ฉฐ, ํ๋๋ ํ๊ท์์ ์ฌ์ฉ๋ ๋ ๋ฆฝ ๋ณ์์ ์ p์ ์ํด, ๋ค๋ฅธ ํ๋๋ ํ๋ณธ ํฌ๊ธฐ n์์ ๋ ๋ฆฝ ๋ณ์์ ์๋ฅผ ๋บ ๊ฐ์ ์ํด ๊ฒฐ์ ๋๋ค. p-๊ฐ ๊ณ์ฐ: ํต๊ณ ์ํํธ์จ์ด๋ ์ฃผ์ด์ง F-ํต๊ณ๋ ๊ฐ๊ณผ ์์ ๋๋ฅผ ์ฌ์ฉํ์ฌ p-๊ฐ์ ๊ณ์ฐํ๋ค. p-๊ฐ์ ํน์ ํ F-ํต๊ณ๋ ๊ฐ์ด ๊ด์ธก๋ ํ๋ฅ ์ ๋ํ๋ด๋ฉฐ, ์ด ๊ฐ์ด ๋งค์ฐ ๋ฎ๋ค๋ฉด (์ผ๋ฐ์ ์ผ๋ก 0.05 ๋๋ 0.01 ๋ฏธ๋ง) ์ฐ์ฐํ ๋ฐ์ํ๋ค๊ณ ๋ณด๊ธฐ ์ด..
Bias(ํธํฅ) : Gap between the real problem and our model / The expected error created by using a model to apporoximate a real world function/relationship. ๋ชจ๋ธ์ ํตํด ์ป์ ์์ธก๊ฐ๊ณผ ์ค์ ์ ๋ต๊ณผ์ ์ฐจ์ด์ ํ๊ท f hat(x) : predicted value f(x) : true value Bias[^f(x)]=E[^f(x)−f(x)] Variance(๋ถ์ฐ) : change for different training data sets / The amount our predicted values would change if we had a differenct training dataset. It..
Criteria Logistic Regression Naive Bayes Classifier Linear Discriminant Analysis (LDA) Quadratic Discriminant Analysis (QDA) Model Type Parametric Parametric Parametric Parametric Assumption about Data Distribution None on distribution, assumes a linear relationship between log odds and features Assumes independence between features, with specific distribution per class Assumes Gaussian distribu..
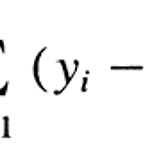
Assessing Model Accuracy So many machine learning methods! • A single best method for all data sets? Nope! • One method may work best on a particular data set. • But, some other method may work better on a similar but different data set How to compare Methods? • Given a set of data, which method will produce the best result? • In other words, how to compare different learning methods? Measuring ..
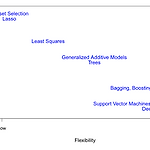
Key terms Y = f(x1, x2, x3) - want to improve sales (Y) of a product -> Y: output variable, dependent variable -control adveritsing budgets : sns(x1), streaming(x2), flier(x3) ->x1, x2, x3 : input variables, independent variables, predictors Key questions 1) What is the relationship between x1, x2, x3 and Y? -> learning 2) How accurately can we predict Y from x1, x2, x3? -> prediction data -- (l..
statistical learning supervised/ unsupervised supervised : given data (x,y), build a model, a mapping Y(input) ~ f(x)(output) - use the model f to predict Y for unknown inputs X' find relationship between factors based on data ex) based on various values year, age, gender ... => wage consider outlier (์ด์์น) https://en.wikipedia.org/wiki/Outlier Outlier - Wikipedia From Wikipedia, the free encyclo..
1. Input Layer:Purpose: It receives the input data and acts as a gateway to funnel the data into the network. Nodes: The number of nodes in the input layer typically matches the number of input features in the dataset. For instance, for an image that's 28x28 pixels (like those in the MNIST dataset), you'd have 784 input nodes. 2. Output Layer:Purpose: This is where the network provides its pred..
Limitations of the Simple Perceptron The primary limitation of a simple perceptron is its inability to handle data that isn't linearly separable. This means that if you can't draw a straight line (in two dimensions, or a hyper-plane in higher dimensions) to separate the classes, the perceptron won't be able to classify the data correctly. In the context of logical operations, a perceptron can mo..
Major Topics of NN NN or Neuron itself At its core, a neural network is comprised of nodes or "neurons", which are inspired by the biological neurons found in our brains. These artificial neurons receive inputs, process them (via weights and biases) and produce an output. Architectures FNN (Feedforward Neural Network): Data flows in one direction, from input to output. It consists of an input la..